What makes you ecologically responsible nowadays? Well, there are some no-brainers most of us have assimilated, like turning off the tap while brushing your teeth, buying locally grown vegetables and fruits, or using a bike or public transportation instead of a car (even if it’s electric). But that’s just the tip of the iceberg. There is an immense body of carbon emissions floating deep under the water line that we unleash every time we interact with the digital world. The surge of generative AI and the frenzy with which it has been hyped as a universal problem solver is dramatically deteriorating the carbon footprint of the whole ICT (Information and Communications Technology) industry. Let’s try to quantify this and see what each of us can undertake to curb the energy consumption of AI.
Beware of the carbon footprint of your digital life
“How bad are bananas?” In this bestseller first published in 2009, Mike Berners-Lee nurtured the ambition to educate us about the carbon footprint of… well, everything in our daily lives. In 2020, given the pace at which digital disruptions were happening, he published an update integrating major innovations like Bitcoin and the Cloud. Since then, calculating the carbon footprint of human activities has become a favorite discipline of researchers, climate activists and journalists alike. A plethora of not always congruous figures are vehiculated throughout the Web – which, ironically, does not serve the purpose of reducing carbon emissions.
Here is a comparison of the CO2 emissions of our digital activities, gleaned from several sources:
Activity | CO2 emissions | Unit |
Browsing the internet | 0,8g | Per page |
Sending a request to ChatGPT | 4,32g | Per request |
Sending an email with attachment | 50g | Per email |
TikTok consumption | 158g | Per hour |
Video conference between 2 persons | 270g | Per hour |
Video streaming on Netflix | 432g – 1.682g | Per hour |
The carbon emissions of calling ChatGPT compared to sending an email or watching a video do not frighten you? Well, they should. It’s all about the sheer quantity of interactions happening every single minute with a large language model. Continue reading!
In the context of global warming and what we know of the energy greediness of AI, estimating the carbon footprint of AI should appear at the top of the agenda of Google, Meta and the other tech giants developing large language models or integrating them in their products. But it doesn’t. The tech giants do not share figures about the carbon footprint of training and using LLMs, and there are no standardized methods of measuring the emissions of AI either. Interestingly, we owe the first findings about the carbon footprint of LLMs to an AI startup called Hugging Face. Their team has researched both the emissions produced by training an LLM and by running it for different tasks like text classification, summarization and image generation.
The first interesting finding is that your AI carbon footprint may vary a lot depending on your location: it will be much lower in a country like France using a relatively clean power grid, compared to other regions like parts of the US or China still largely dependent on fossil energy. Including the emissions from manufacturing the computing infrastructure, Hugging Face’s researchers estimated the total emissions of their own LLM, Bloom, which was trained on a supercomputer in France, to 50 tons of CO2 – significantly less than similar LLMs such as Meta’s OPT (75 tons) and OpenAI’s GPT-3 (500 tons).
Training an LLM | CO2 emissions |
Bloom | 50 tons |
OPT | 75 tons |
GPT-3 | 500 tons |
We all knew that training an LLM consumes a lot of energy (see previous blog posts). The interesting discovery here is that where the LLM is trained has a huge impact on its carbon footprint. The biggest surprise from this research, however, comes as a knock-out: Training the LLM does not make for the biggest part of its carbon footprint. Running it is the real energy guzzler.
Looking at the emissions for single tasks does not look frightening though – except for image generation whose emissions skyrocket to 1.8kg per 1.000 requests, all other tasks incl. text summarization remain well below 100g per 1.000 requests:
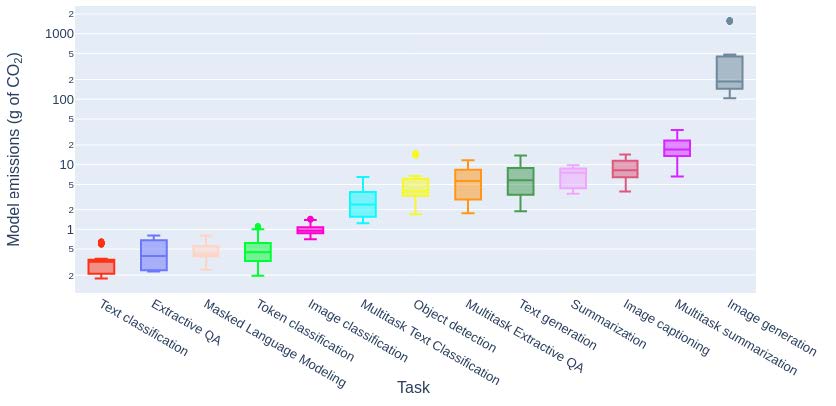
Copyright: Luccioni, Jernite and Strubell – Power Hungry Processing: Watts Driving the cost of AI development
Compared to 500 tons CO2 for training a single model, 1,8kg for generating an image does not sound like a threat to our climate. The problem here is not the seemingly low level of emission for each single interaction with a generative AI. It is the fact that all major tech companies integrate generative AI capabilities in their systems and that billions of interactions are done every day by the users of Microsoft, Meta, Adobe, Salesforce and the likes. Whether you write an email, draft a presentation, summarize a call, or create a social media post, now your favorite applications give you a direct access to large language models for any other task. The more a LLM is used, the more likely the carbon footprint from inferences will be higher than from training. For popular models like ChatGPT with 10 millions of users every day, the model’s usage emissions are estimated to have exceeded the training emissions within a couple of weeks!
Is sending less requests to ChatGPT and refraining from generating yet another funny image for your social media post the solution to the exploding AI carbon footprint? Certainly, as it would be wise to replace attachments with links in your emails or limit the number of videos you upload/watch on TikTok.
But the lion share of AI carbon emissions belongs to the companies training or leveraging LLMs in their daily operations. If your organization is experimenting with generative AI, here is what you can do to keep your carbon emissions as low as possible.
How to surf the AI wave and preserve the environment
Use a large language model only if it brings a significant benefit
Very often, LLMs are an overkill. For example, developing an application to search your intranet or classify your emails does not require an LLM. Such applications can be built in-house with pre-trained models, or simply bought from specialized vendors. Also think twice before switching to an LLM just to get 1 or 2% more accuracy. These extra points probably won’t justify to use 2 or 3 times more power, will they?
Fine-tune existing models, rather than train new models from scratch
If you really think you need your own generative AI model, take an existing one and customize it to your specific domain. Look at open-source models, which your AI experts and data scientists can fine-tune. Researchers found out that classifying movie reviews with an LLM consumes 30 times more energy than with a smaller model fine-tuned for this task. The reason is that LLMs try to perform several tasks like generate, classify, and summarize text, instead of just one task.
Assess the eco-friendliness of your cloud provider or data center
Find out where your computing infrastructure is getting its power from: What is the proportion of renewable energy versus fossil fuels? Deploying models in a region with a higher share of clean power can reduce operational emissions by 75%, as shown by this practice.
Track the CO2 emission of your AI activity – and integrate it in your reporting
There are more and more tools to help you monitor the emissions of your AI activities. They can be included in your code at runtime to estimate your emissions and facilitate reporting. For more information, visit CodeCarbon, Green algorithms, or ML CO2 Impact.
I have a dream: Sustainable AI for everyone
As long as reporting about the AI carbon footprint remains optional, as long as no international standards are established to structure and compare the different estimations of CO2 emissions, reducing the environmental impact of AI remains a dream. But you and I can contribute to making this dream a reality. Today, I have refrained from sending a dozen requests to an AI image generator to get a striking illustration for this post – I chose instead a stock photo. Tomorrow, I will talk with a large US insurer who has decided to use Cortical.io SemanticPro for extracting and classifying information from documents – a system that needs much less computing power than LLMs to process documents. What about you?